Kaggle Digit Recognizer series
The initial results (previous post) for the digit classifier were coming in with an accuracy 4 points below the Kaggle-provided sample solutions. This was with only two naive attempts. First was a neural net with a 25-node hidden layer trained over 50 iterations. Next was a net with a 300-node hidden layer trained for 5000 iterations. Some improvement may be gained by tuning the regularization lambda. However no training data subsetting and cross validation has been done yet towards this goal.
It seemed reasonable (and interesting!) to modify the code to allow a second hidden node layer in pursuit of better results. Where to start? The new code will be cloned from what is already working. The single hidden layer functions will remain intact to allow easy side-by-side testing of solutions. The cost function clearly needs to change so nnCostFunction2H()
will be used for that. I’m adopting a 2H suffix for functions which support the dual hidden layer network model.
I like the confidence that comes from checking the cost function’s gradients against computed numerical gradients so there will be a checkNNGradients2H()
as well.
A predict2H()
will be needed too. It would be preferable to have all the training and test data prediction code together in a single function. But at this point in development I would rather have the single and dual hidden layer top level orchestration code in separate files to avoid if-then toxemia in getting all the bits right. Therefore we’ll have trainNN2H.m
and runNN2H.m
as top level scripts for training the net and producing predicted classifications for submission to Kaggle.
So the changes are really not that extensive. They must be precise though, no room to get sloppy if the vectorized code is expected to work properly. The part of the existing code that was bothering me most deals with reconstructing the Theta matrices. I think there is too much math going on as parameters to the reshape()
function calls. I find code like this hard to read and frightening to consider extending:
% Obtain Theta1 and Theta2 back from nn_params
Theta1 = reshape(nn_params(1:hidden_layer_size * (input_layer_size + 1)), ...
hidden_layer_size, (input_layer_size + 1));
Theta2 = reshape(nn_params((1 + (hidden_layer_size * (input_layer_size + 1))):end), ...
num_labels, (hidden_layer_size + 1));
I need a picture of what is happening here!
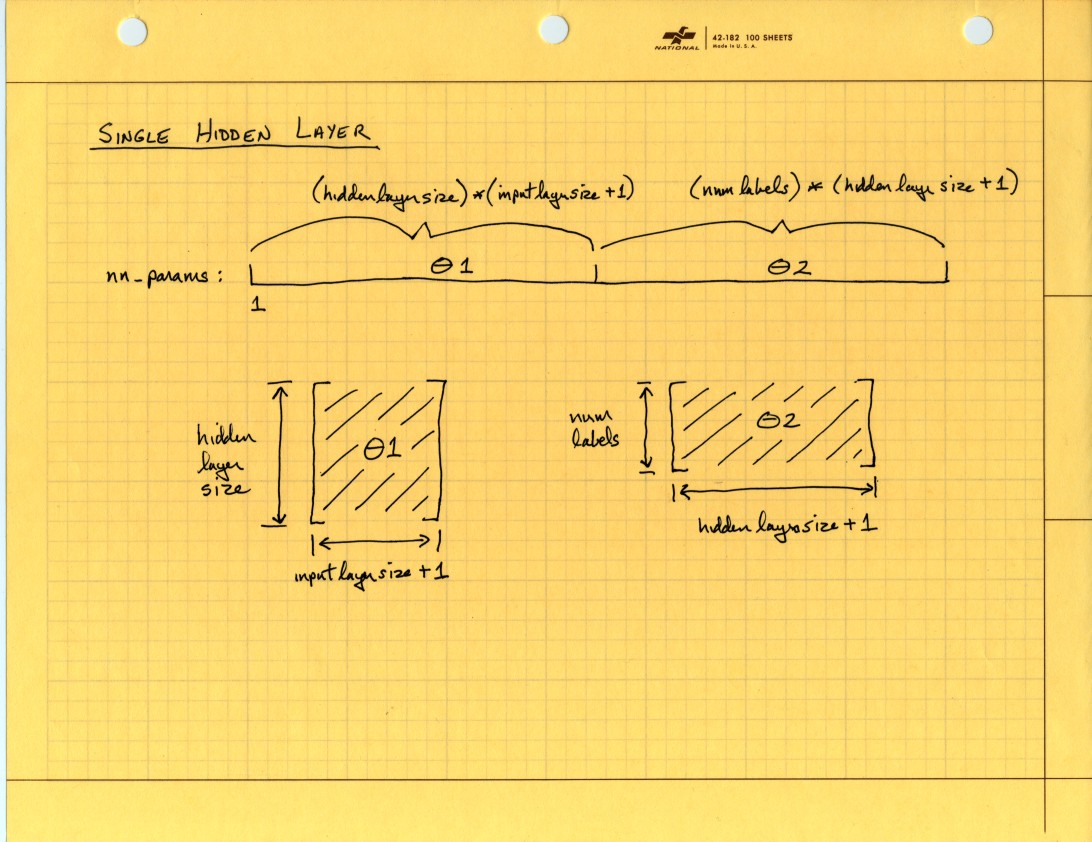
So then for two hidden layers Theta recovery will look like this:

And from that picture I can see a pattern of how the Theta dimensions progress giving me a pretty good idea how to proceed if I want to parameterize the hidden layer depth. Also, the Theta recovery is done in at least two places in the existing code so I’ve replaced it with a function:
function [Theta1 Theta2 Theta3] = reshapeTheta2H(nn_params, ...
input_layer_size, ...
hidden_layer_size, ...
hidden_layer2_size, ...
num_labels)
%reshapeTheta2H Recovers Theta matrices for 2 hidden layer NN from flattened vector
Theta1_size = hidden_layer_size * (input_layer_size + 1);
Theta2_size = hidden_layer2_size * (hidden_layer_size + 1);
Theta3_size = num_labels * (hidden_layer2_size + 1);
Theta1_start = 1;
Theta2_start = Theta1_size + 1;
Theta3_start = Theta1_size + Theta2_size + 1;
Theta1 = reshape(nn_params(Theta1_start : Theta1_size), ...
hidden_layer_size, (input_layer_size + 1));
Theta2 = reshape(nn_params(Theta2_start : (Theta1_size + Theta2_size)), ...
hidden_layer2_size, (hidden_layer_size + 1));
Theta3 = reshape(nn_params(Theta3_start : end), ...
num_labels, (hidden_layer2_size + 1));
end
Now another naive test run, no regularization tuning yet. This neural net will use 350 nodes in the first hidden layer, 100 nodes in the second hidden layer, lambda = 1, and 500 training iterations. The full training set of 42,000 samples will be used.
But no! Octave appears to be failing on the first iteration inside the fmincg()
optimization function. The error message is not very helpful. It complains of being out of memory or an index exceeding its range. No line number given. This needs investigation but not right now, I’d really like to see some results.
Cutting down the training set size by 20% to 33,600 samples works with no complaints. Run time is just under 3600 seconds (1 hour). Final iteration (500) cost function value is 0.0596 and self-classified accuracy is 99.821%. The Kaggle submission for this net scored 96.486% accuracy.
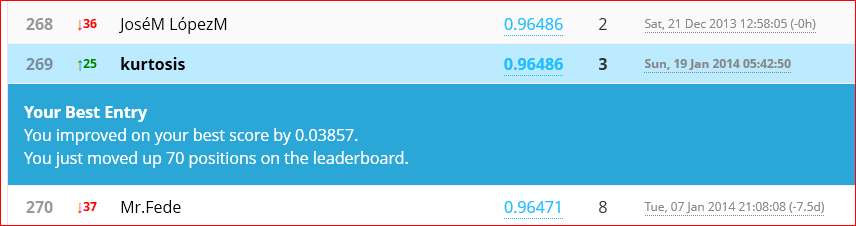
That is an improvement, but not so much. It is still under the Kaggle sample solution performance but getting closer. Now it’s time to put the science in Data Science. Next to-do is cross validation to find a proper lambda. And next after that will be examining training vs cross validation error rates over a range of sample set sizes. This should tell if the model is having trouble with high bias or high variance.